Validation accuracy vs Testing accuracyInformation on how value of k in k-fold cross-validation affects resulting accuraciesEstimating the variance of a bootstrap aggregator performance?Inconsistency in cross-validation resultsCross-validation including training, validation, and testing. Why do we need three subsets?My Test accuracy is pretty bad compared to cross-validation accuracyBetter accuracy with validation set than test setFeature selection: is nested cross-validation needed?10-fold cross validation, why having a validation set?Bias-Variance terminology for loss functions in ML vs cross-validation — different things?Is cross-validation better/worse than a third holdout set?
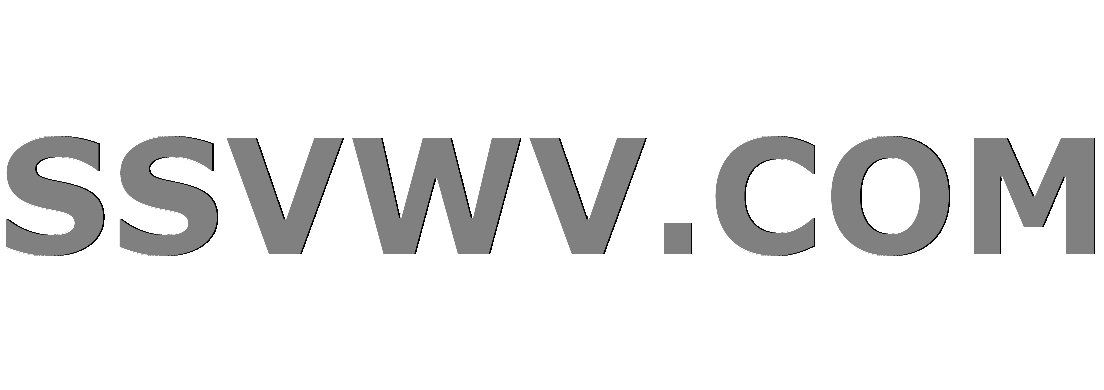
Multi tool use
Why is my log file so massive? 22gb. I am running log backups
Shall I use personal or official e-mail account when registering to external websites for work purpose?
"My colleague's body is amazing"
Check if two datetimes are between two others
Does it makes sense to buy a new cycle to learn riding?
Lied on resume at previous job
Can I legally use front facing blue light in the UK?
If a centaur druid Wild Shapes into a Giant Elk, do their Charge features stack?
Finding files for which a command fails
Typesetting a double Over Dot on top of a symbol
What to wear for invited talk in Canada
Is this food a bread or a loaf?
Can a planet have a different gravitational pull depending on its location in orbit around its sun?
Need help identifying/translating a plaque in Tangier, Morocco
Prime joint compound before latex paint?
What is the offset in a seaplane's hull?
extract characters between two commas?
When blogging recipes, how can I support both readers who want the narrative/journey and ones who want the printer-friendly recipe?
Pristine Bit Checking
A poker game description that does not feel gimmicky
I’m planning on buying a laser printer but concerned about the life cycle of toner in the machine
Information to fellow intern about hiring?
How could a lack of term limits lead to a "dictatorship?"
How did the USSR manage to innovate in an environment characterized by government censorship and high bureaucracy?
Validation accuracy vs Testing accuracy
Information on how value of k in k-fold cross-validation affects resulting accuraciesEstimating the variance of a bootstrap aggregator performance?Inconsistency in cross-validation resultsCross-validation including training, validation, and testing. Why do we need three subsets?My Test accuracy is pretty bad compared to cross-validation accuracyBetter accuracy with validation set than test setFeature selection: is nested cross-validation needed?10-fold cross validation, why having a validation set?Bias-Variance terminology for loss functions in ML vs cross-validation — different things?Is cross-validation better/worse than a third holdout set?
.everyoneloves__top-leaderboard:empty,.everyoneloves__mid-leaderboard:empty,.everyoneloves__bot-mid-leaderboard:empty margin-bottom:0;
$begingroup$
I am trying to get my head straight on terminology which appears confusing. I know there are three 'splits' of data used in Machine learning models.:
- Training Data - Train the model
- Validation Data - Cross validation for model selection
- Testing Data - Test the generalisation error.
Now, as far as I am aware, the validation data is not always used as one can use k-fold cross-validation, reducing the need to further reduce ones dataset. The results of which are known as the validation accuracy. Then once the best model is selected, the model is tested on a 33% split from the initial data set (which has not been used to train). The results of this would be the testing accuracy?
Is this the right way around? or is vice versa? I am finding conflicting terminology used online! I am trying to find some explanations why my validation error is larger than my testing error, but before I find a solution, i would like to get my terminology correct.
Thanks.
machine-learning
$endgroup$
add a comment |
$begingroup$
I am trying to get my head straight on terminology which appears confusing. I know there are three 'splits' of data used in Machine learning models.:
- Training Data - Train the model
- Validation Data - Cross validation for model selection
- Testing Data - Test the generalisation error.
Now, as far as I am aware, the validation data is not always used as one can use k-fold cross-validation, reducing the need to further reduce ones dataset. The results of which are known as the validation accuracy. Then once the best model is selected, the model is tested on a 33% split from the initial data set (which has not been used to train). The results of this would be the testing accuracy?
Is this the right way around? or is vice versa? I am finding conflicting terminology used online! I am trying to find some explanations why my validation error is larger than my testing error, but before I find a solution, i would like to get my terminology correct.
Thanks.
machine-learning
$endgroup$
add a comment |
$begingroup$
I am trying to get my head straight on terminology which appears confusing. I know there are three 'splits' of data used in Machine learning models.:
- Training Data - Train the model
- Validation Data - Cross validation for model selection
- Testing Data - Test the generalisation error.
Now, as far as I am aware, the validation data is not always used as one can use k-fold cross-validation, reducing the need to further reduce ones dataset. The results of which are known as the validation accuracy. Then once the best model is selected, the model is tested on a 33% split from the initial data set (which has not been used to train). The results of this would be the testing accuracy?
Is this the right way around? or is vice versa? I am finding conflicting terminology used online! I am trying to find some explanations why my validation error is larger than my testing error, but before I find a solution, i would like to get my terminology correct.
Thanks.
machine-learning
$endgroup$
I am trying to get my head straight on terminology which appears confusing. I know there are three 'splits' of data used in Machine learning models.:
- Training Data - Train the model
- Validation Data - Cross validation for model selection
- Testing Data - Test the generalisation error.
Now, as far as I am aware, the validation data is not always used as one can use k-fold cross-validation, reducing the need to further reduce ones dataset. The results of which are known as the validation accuracy. Then once the best model is selected, the model is tested on a 33% split from the initial data set (which has not been used to train). The results of this would be the testing accuracy?
Is this the right way around? or is vice versa? I am finding conflicting terminology used online! I am trying to find some explanations why my validation error is larger than my testing error, but before I find a solution, i would like to get my terminology correct.
Thanks.
machine-learning
machine-learning
asked yesterday
BillyJo_ramblerBillyJo_rambler
296
296
add a comment |
add a comment |
2 Answers
2
active
oldest
votes
$begingroup$
There isn't a standard terminology in this context (and I have seen long discussions and debates regarding this topic), so I completely understand you, but you should get used to different terminology (and assume that terminology might not be consistent or it change across sources).
I would like to point out a few things:
I have never seen people use the expression "validation accuracy" (or dataset) to refer to the test accuracy (or dataset), but I have seen people use the term "test accuracy" (or dataset) to refer to the validation accuracy (or dataset). In other words, the test (or testing) accuracy often refers to the validation accuracy, that is, the accuracy you calculate on the data set you do not use for training, but you use (during the training process) for validating (or "testing") the generalisation ability of your model or for "early stopping".
In k-fold cross-validation, people usually only mention two datasets: training and testing (or validation).
k-fold cross-validation is just a way of validating the model on different subsets of the data. This can be done for several reasons. For example, you have a small amount of data, so your validation (and training) dataset is quite small, so you want to have a better understanding of the model's generalisation ability by validating it on several subsets of the whole dataset.
You should likely have a separate (from the validation dataset) dataset for testing, because the validation dataset can be used for early stopping, so, in a certain way, it is dependent on the training process
I would suggest to use the following terminology
- Training dataset: the data used to fit the model.
- Validation dataset: the data used to validate the generalisation ability of the model or for early stopping, during the training process.
- Testing dataset: the data used to for other purposes other than training and validating.
Note that some of these datasets might overlap, but this might almost never be a good thing (if you have enough data).
$endgroup$
$begingroup$
If the testing dataset overlaps with either of the others, it is definitely not a good thing. The test accuracy must measure performance on unseen data. If any part of training saw the data, then it isn't test data, and representing it as such is dishonest. Allowing the validation set to overlap with the training set isn't dishonest, but it probably won't accomplish its task as well. (e.g., if you're doing early stopping, and your validation set and training sets overlap, overfitting may occur and not be detected.)
$endgroup$
– Ray
yesterday
$begingroup$
@Ray I didn't say it is a good thing. Indeed, see my point "You should likely have a separate (from the validation dataset) dataset for testing...".
$endgroup$
– nbro
yesterday
$begingroup$
You said "If that's a 'good' thing or not, it's another question." I suspected from the rest that you understood the problems that that overlap would cause, but the problems with that should be made very clear, since contaminating your test data with training samples completely ruins its value.
$endgroup$
– Ray
yesterday
$begingroup$
@Ray I wanted more to refer to the overlap between the training and validation datasets. Anyway, I think it's good that you wanted to clarify or emphasise this point. I edited my answer to emphasise this point.
$endgroup$
– nbro
yesterday
add a comment |
$begingroup$
@nbro's answer is complete. I just add a couple of explanations to supplement. In more traditional textbooks data is often partitioned into two sets: training and test. In recent years, with more complex models and increasing need for model selection, development sets or validations sets are also considered. Devel/validation should have no overlap with the test set or the reporting accuracy/ error evaluation is not valid. In the modern setting: the model is trained on the training set, tested on the validation set to see if it is a good fit, possibly model is tweaked and trained again and validated again for multiple times. When the final model is selected, the testing set is used to calculate accuracy, error reports. The important thing is that the test set is only touched once.
New contributor
user3089485 is a new contributor to this site. Take care in asking for clarification, commenting, and answering.
Check out our Code of Conduct.
$endgroup$
add a comment |
Your Answer
StackExchange.ifUsing("editor", function ()
return StackExchange.using("mathjaxEditing", function ()
StackExchange.MarkdownEditor.creationCallbacks.add(function (editor, postfix)
StackExchange.mathjaxEditing.prepareWmdForMathJax(editor, postfix, [["$", "$"], ["\\(","\\)"]]);
);
);
, "mathjax-editing");
StackExchange.ready(function()
var channelOptions =
tags: "".split(" "),
id: "65"
;
initTagRenderer("".split(" "), "".split(" "), channelOptions);
StackExchange.using("externalEditor", function()
// Have to fire editor after snippets, if snippets enabled
if (StackExchange.settings.snippets.snippetsEnabled)
StackExchange.using("snippets", function()
createEditor();
);
else
createEditor();
);
function createEditor()
StackExchange.prepareEditor(
heartbeatType: 'answer',
autoActivateHeartbeat: false,
convertImagesToLinks: false,
noModals: true,
showLowRepImageUploadWarning: true,
reputationToPostImages: null,
bindNavPrevention: true,
postfix: "",
imageUploader:
brandingHtml: "Powered by u003ca class="icon-imgur-white" href="https://imgur.com/"u003eu003c/au003e",
contentPolicyHtml: "User contributions licensed under u003ca href="https://creativecommons.org/licenses/by-sa/3.0/"u003ecc by-sa 3.0 with attribution requiredu003c/au003e u003ca href="https://stackoverflow.com/legal/content-policy"u003e(content policy)u003c/au003e",
allowUrls: true
,
onDemand: true,
discardSelector: ".discard-answer"
,immediatelyShowMarkdownHelp:true
);
);
Sign up or log in
StackExchange.ready(function ()
StackExchange.helpers.onClickDraftSave('#login-link');
);
Sign up using Google
Sign up using Facebook
Sign up using Email and Password
Post as a guest
Required, but never shown
StackExchange.ready(
function ()
StackExchange.openid.initPostLogin('.new-post-login', 'https%3a%2f%2fstats.stackexchange.com%2fquestions%2f401696%2fvalidation-accuracy-vs-testing-accuracy%23new-answer', 'question_page');
);
Post as a guest
Required, but never shown
2 Answers
2
active
oldest
votes
2 Answers
2
active
oldest
votes
active
oldest
votes
active
oldest
votes
$begingroup$
There isn't a standard terminology in this context (and I have seen long discussions and debates regarding this topic), so I completely understand you, but you should get used to different terminology (and assume that terminology might not be consistent or it change across sources).
I would like to point out a few things:
I have never seen people use the expression "validation accuracy" (or dataset) to refer to the test accuracy (or dataset), but I have seen people use the term "test accuracy" (or dataset) to refer to the validation accuracy (or dataset). In other words, the test (or testing) accuracy often refers to the validation accuracy, that is, the accuracy you calculate on the data set you do not use for training, but you use (during the training process) for validating (or "testing") the generalisation ability of your model or for "early stopping".
In k-fold cross-validation, people usually only mention two datasets: training and testing (or validation).
k-fold cross-validation is just a way of validating the model on different subsets of the data. This can be done for several reasons. For example, you have a small amount of data, so your validation (and training) dataset is quite small, so you want to have a better understanding of the model's generalisation ability by validating it on several subsets of the whole dataset.
You should likely have a separate (from the validation dataset) dataset for testing, because the validation dataset can be used for early stopping, so, in a certain way, it is dependent on the training process
I would suggest to use the following terminology
- Training dataset: the data used to fit the model.
- Validation dataset: the data used to validate the generalisation ability of the model or for early stopping, during the training process.
- Testing dataset: the data used to for other purposes other than training and validating.
Note that some of these datasets might overlap, but this might almost never be a good thing (if you have enough data).
$endgroup$
$begingroup$
If the testing dataset overlaps with either of the others, it is definitely not a good thing. The test accuracy must measure performance on unseen data. If any part of training saw the data, then it isn't test data, and representing it as such is dishonest. Allowing the validation set to overlap with the training set isn't dishonest, but it probably won't accomplish its task as well. (e.g., if you're doing early stopping, and your validation set and training sets overlap, overfitting may occur and not be detected.)
$endgroup$
– Ray
yesterday
$begingroup$
@Ray I didn't say it is a good thing. Indeed, see my point "You should likely have a separate (from the validation dataset) dataset for testing...".
$endgroup$
– nbro
yesterday
$begingroup$
You said "If that's a 'good' thing or not, it's another question." I suspected from the rest that you understood the problems that that overlap would cause, but the problems with that should be made very clear, since contaminating your test data with training samples completely ruins its value.
$endgroup$
– Ray
yesterday
$begingroup$
@Ray I wanted more to refer to the overlap between the training and validation datasets. Anyway, I think it's good that you wanted to clarify or emphasise this point. I edited my answer to emphasise this point.
$endgroup$
– nbro
yesterday
add a comment |
$begingroup$
There isn't a standard terminology in this context (and I have seen long discussions and debates regarding this topic), so I completely understand you, but you should get used to different terminology (and assume that terminology might not be consistent or it change across sources).
I would like to point out a few things:
I have never seen people use the expression "validation accuracy" (or dataset) to refer to the test accuracy (or dataset), but I have seen people use the term "test accuracy" (or dataset) to refer to the validation accuracy (or dataset). In other words, the test (or testing) accuracy often refers to the validation accuracy, that is, the accuracy you calculate on the data set you do not use for training, but you use (during the training process) for validating (or "testing") the generalisation ability of your model or for "early stopping".
In k-fold cross-validation, people usually only mention two datasets: training and testing (or validation).
k-fold cross-validation is just a way of validating the model on different subsets of the data. This can be done for several reasons. For example, you have a small amount of data, so your validation (and training) dataset is quite small, so you want to have a better understanding of the model's generalisation ability by validating it on several subsets of the whole dataset.
You should likely have a separate (from the validation dataset) dataset for testing, because the validation dataset can be used for early stopping, so, in a certain way, it is dependent on the training process
I would suggest to use the following terminology
- Training dataset: the data used to fit the model.
- Validation dataset: the data used to validate the generalisation ability of the model or for early stopping, during the training process.
- Testing dataset: the data used to for other purposes other than training and validating.
Note that some of these datasets might overlap, but this might almost never be a good thing (if you have enough data).
$endgroup$
$begingroup$
If the testing dataset overlaps with either of the others, it is definitely not a good thing. The test accuracy must measure performance on unseen data. If any part of training saw the data, then it isn't test data, and representing it as such is dishonest. Allowing the validation set to overlap with the training set isn't dishonest, but it probably won't accomplish its task as well. (e.g., if you're doing early stopping, and your validation set and training sets overlap, overfitting may occur and not be detected.)
$endgroup$
– Ray
yesterday
$begingroup$
@Ray I didn't say it is a good thing. Indeed, see my point "You should likely have a separate (from the validation dataset) dataset for testing...".
$endgroup$
– nbro
yesterday
$begingroup$
You said "If that's a 'good' thing or not, it's another question." I suspected from the rest that you understood the problems that that overlap would cause, but the problems with that should be made very clear, since contaminating your test data with training samples completely ruins its value.
$endgroup$
– Ray
yesterday
$begingroup$
@Ray I wanted more to refer to the overlap between the training and validation datasets. Anyway, I think it's good that you wanted to clarify or emphasise this point. I edited my answer to emphasise this point.
$endgroup$
– nbro
yesterday
add a comment |
$begingroup$
There isn't a standard terminology in this context (and I have seen long discussions and debates regarding this topic), so I completely understand you, but you should get used to different terminology (and assume that terminology might not be consistent or it change across sources).
I would like to point out a few things:
I have never seen people use the expression "validation accuracy" (or dataset) to refer to the test accuracy (or dataset), but I have seen people use the term "test accuracy" (or dataset) to refer to the validation accuracy (or dataset). In other words, the test (or testing) accuracy often refers to the validation accuracy, that is, the accuracy you calculate on the data set you do not use for training, but you use (during the training process) for validating (or "testing") the generalisation ability of your model or for "early stopping".
In k-fold cross-validation, people usually only mention two datasets: training and testing (or validation).
k-fold cross-validation is just a way of validating the model on different subsets of the data. This can be done for several reasons. For example, you have a small amount of data, so your validation (and training) dataset is quite small, so you want to have a better understanding of the model's generalisation ability by validating it on several subsets of the whole dataset.
You should likely have a separate (from the validation dataset) dataset for testing, because the validation dataset can be used for early stopping, so, in a certain way, it is dependent on the training process
I would suggest to use the following terminology
- Training dataset: the data used to fit the model.
- Validation dataset: the data used to validate the generalisation ability of the model or for early stopping, during the training process.
- Testing dataset: the data used to for other purposes other than training and validating.
Note that some of these datasets might overlap, but this might almost never be a good thing (if you have enough data).
$endgroup$
There isn't a standard terminology in this context (and I have seen long discussions and debates regarding this topic), so I completely understand you, but you should get used to different terminology (and assume that terminology might not be consistent or it change across sources).
I would like to point out a few things:
I have never seen people use the expression "validation accuracy" (or dataset) to refer to the test accuracy (or dataset), but I have seen people use the term "test accuracy" (or dataset) to refer to the validation accuracy (or dataset). In other words, the test (or testing) accuracy often refers to the validation accuracy, that is, the accuracy you calculate on the data set you do not use for training, but you use (during the training process) for validating (or "testing") the generalisation ability of your model or for "early stopping".
In k-fold cross-validation, people usually only mention two datasets: training and testing (or validation).
k-fold cross-validation is just a way of validating the model on different subsets of the data. This can be done for several reasons. For example, you have a small amount of data, so your validation (and training) dataset is quite small, so you want to have a better understanding of the model's generalisation ability by validating it on several subsets of the whole dataset.
You should likely have a separate (from the validation dataset) dataset for testing, because the validation dataset can be used for early stopping, so, in a certain way, it is dependent on the training process
I would suggest to use the following terminology
- Training dataset: the data used to fit the model.
- Validation dataset: the data used to validate the generalisation ability of the model or for early stopping, during the training process.
- Testing dataset: the data used to for other purposes other than training and validating.
Note that some of these datasets might overlap, but this might almost never be a good thing (if you have enough data).
edited yesterday
answered yesterday


nbronbro
8111023
8111023
$begingroup$
If the testing dataset overlaps with either of the others, it is definitely not a good thing. The test accuracy must measure performance on unseen data. If any part of training saw the data, then it isn't test data, and representing it as such is dishonest. Allowing the validation set to overlap with the training set isn't dishonest, but it probably won't accomplish its task as well. (e.g., if you're doing early stopping, and your validation set and training sets overlap, overfitting may occur and not be detected.)
$endgroup$
– Ray
yesterday
$begingroup$
@Ray I didn't say it is a good thing. Indeed, see my point "You should likely have a separate (from the validation dataset) dataset for testing...".
$endgroup$
– nbro
yesterday
$begingroup$
You said "If that's a 'good' thing or not, it's another question." I suspected from the rest that you understood the problems that that overlap would cause, but the problems with that should be made very clear, since contaminating your test data with training samples completely ruins its value.
$endgroup$
– Ray
yesterday
$begingroup$
@Ray I wanted more to refer to the overlap between the training and validation datasets. Anyway, I think it's good that you wanted to clarify or emphasise this point. I edited my answer to emphasise this point.
$endgroup$
– nbro
yesterday
add a comment |
$begingroup$
If the testing dataset overlaps with either of the others, it is definitely not a good thing. The test accuracy must measure performance on unseen data. If any part of training saw the data, then it isn't test data, and representing it as such is dishonest. Allowing the validation set to overlap with the training set isn't dishonest, but it probably won't accomplish its task as well. (e.g., if you're doing early stopping, and your validation set and training sets overlap, overfitting may occur and not be detected.)
$endgroup$
– Ray
yesterday
$begingroup$
@Ray I didn't say it is a good thing. Indeed, see my point "You should likely have a separate (from the validation dataset) dataset for testing...".
$endgroup$
– nbro
yesterday
$begingroup$
You said "If that's a 'good' thing or not, it's another question." I suspected from the rest that you understood the problems that that overlap would cause, but the problems with that should be made very clear, since contaminating your test data with training samples completely ruins its value.
$endgroup$
– Ray
yesterday
$begingroup$
@Ray I wanted more to refer to the overlap between the training and validation datasets. Anyway, I think it's good that you wanted to clarify or emphasise this point. I edited my answer to emphasise this point.
$endgroup$
– nbro
yesterday
$begingroup$
If the testing dataset overlaps with either of the others, it is definitely not a good thing. The test accuracy must measure performance on unseen data. If any part of training saw the data, then it isn't test data, and representing it as such is dishonest. Allowing the validation set to overlap with the training set isn't dishonest, but it probably won't accomplish its task as well. (e.g., if you're doing early stopping, and your validation set and training sets overlap, overfitting may occur and not be detected.)
$endgroup$
– Ray
yesterday
$begingroup$
If the testing dataset overlaps with either of the others, it is definitely not a good thing. The test accuracy must measure performance on unseen data. If any part of training saw the data, then it isn't test data, and representing it as such is dishonest. Allowing the validation set to overlap with the training set isn't dishonest, but it probably won't accomplish its task as well. (e.g., if you're doing early stopping, and your validation set and training sets overlap, overfitting may occur and not be detected.)
$endgroup$
– Ray
yesterday
$begingroup$
@Ray I didn't say it is a good thing. Indeed, see my point "You should likely have a separate (from the validation dataset) dataset for testing...".
$endgroup$
– nbro
yesterday
$begingroup$
@Ray I didn't say it is a good thing. Indeed, see my point "You should likely have a separate (from the validation dataset) dataset for testing...".
$endgroup$
– nbro
yesterday
$begingroup$
You said "If that's a 'good' thing or not, it's another question." I suspected from the rest that you understood the problems that that overlap would cause, but the problems with that should be made very clear, since contaminating your test data with training samples completely ruins its value.
$endgroup$
– Ray
yesterday
$begingroup$
You said "If that's a 'good' thing or not, it's another question." I suspected from the rest that you understood the problems that that overlap would cause, but the problems with that should be made very clear, since contaminating your test data with training samples completely ruins its value.
$endgroup$
– Ray
yesterday
$begingroup$
@Ray I wanted more to refer to the overlap between the training and validation datasets. Anyway, I think it's good that you wanted to clarify or emphasise this point. I edited my answer to emphasise this point.
$endgroup$
– nbro
yesterday
$begingroup$
@Ray I wanted more to refer to the overlap between the training and validation datasets. Anyway, I think it's good that you wanted to clarify or emphasise this point. I edited my answer to emphasise this point.
$endgroup$
– nbro
yesterday
add a comment |
$begingroup$
@nbro's answer is complete. I just add a couple of explanations to supplement. In more traditional textbooks data is often partitioned into two sets: training and test. In recent years, with more complex models and increasing need for model selection, development sets or validations sets are also considered. Devel/validation should have no overlap with the test set or the reporting accuracy/ error evaluation is not valid. In the modern setting: the model is trained on the training set, tested on the validation set to see if it is a good fit, possibly model is tweaked and trained again and validated again for multiple times. When the final model is selected, the testing set is used to calculate accuracy, error reports. The important thing is that the test set is only touched once.
New contributor
user3089485 is a new contributor to this site. Take care in asking for clarification, commenting, and answering.
Check out our Code of Conduct.
$endgroup$
add a comment |
$begingroup$
@nbro's answer is complete. I just add a couple of explanations to supplement. In more traditional textbooks data is often partitioned into two sets: training and test. In recent years, with more complex models and increasing need for model selection, development sets or validations sets are also considered. Devel/validation should have no overlap with the test set or the reporting accuracy/ error evaluation is not valid. In the modern setting: the model is trained on the training set, tested on the validation set to see if it is a good fit, possibly model is tweaked and trained again and validated again for multiple times. When the final model is selected, the testing set is used to calculate accuracy, error reports. The important thing is that the test set is only touched once.
New contributor
user3089485 is a new contributor to this site. Take care in asking for clarification, commenting, and answering.
Check out our Code of Conduct.
$endgroup$
add a comment |
$begingroup$
@nbro's answer is complete. I just add a couple of explanations to supplement. In more traditional textbooks data is often partitioned into two sets: training and test. In recent years, with more complex models and increasing need for model selection, development sets or validations sets are also considered. Devel/validation should have no overlap with the test set or the reporting accuracy/ error evaluation is not valid. In the modern setting: the model is trained on the training set, tested on the validation set to see if it is a good fit, possibly model is tweaked and trained again and validated again for multiple times. When the final model is selected, the testing set is used to calculate accuracy, error reports. The important thing is that the test set is only touched once.
New contributor
user3089485 is a new contributor to this site. Take care in asking for clarification, commenting, and answering.
Check out our Code of Conduct.
$endgroup$
@nbro's answer is complete. I just add a couple of explanations to supplement. In more traditional textbooks data is often partitioned into two sets: training and test. In recent years, with more complex models and increasing need for model selection, development sets or validations sets are also considered. Devel/validation should have no overlap with the test set or the reporting accuracy/ error evaluation is not valid. In the modern setting: the model is trained on the training set, tested on the validation set to see if it is a good fit, possibly model is tweaked and trained again and validated again for multiple times. When the final model is selected, the testing set is used to calculate accuracy, error reports. The important thing is that the test set is only touched once.
New contributor
user3089485 is a new contributor to this site. Take care in asking for clarification, commenting, and answering.
Check out our Code of Conduct.
New contributor
user3089485 is a new contributor to this site. Take care in asking for clarification, commenting, and answering.
Check out our Code of Conduct.
answered yesterday
user3089485user3089485
162
162
New contributor
user3089485 is a new contributor to this site. Take care in asking for clarification, commenting, and answering.
Check out our Code of Conduct.
New contributor
user3089485 is a new contributor to this site. Take care in asking for clarification, commenting, and answering.
Check out our Code of Conduct.
user3089485 is a new contributor to this site. Take care in asking for clarification, commenting, and answering.
Check out our Code of Conduct.
add a comment |
add a comment |
Thanks for contributing an answer to Cross Validated!
- Please be sure to answer the question. Provide details and share your research!
But avoid …
- Asking for help, clarification, or responding to other answers.
- Making statements based on opinion; back them up with references or personal experience.
Use MathJax to format equations. MathJax reference.
To learn more, see our tips on writing great answers.
Sign up or log in
StackExchange.ready(function ()
StackExchange.helpers.onClickDraftSave('#login-link');
);
Sign up using Google
Sign up using Facebook
Sign up using Email and Password
Post as a guest
Required, but never shown
StackExchange.ready(
function ()
StackExchange.openid.initPostLogin('.new-post-login', 'https%3a%2f%2fstats.stackexchange.com%2fquestions%2f401696%2fvalidation-accuracy-vs-testing-accuracy%23new-answer', 'question_page');
);
Post as a guest
Required, but never shown
Sign up or log in
StackExchange.ready(function ()
StackExchange.helpers.onClickDraftSave('#login-link');
);
Sign up using Google
Sign up using Facebook
Sign up using Email and Password
Post as a guest
Required, but never shown
Sign up or log in
StackExchange.ready(function ()
StackExchange.helpers.onClickDraftSave('#login-link');
);
Sign up using Google
Sign up using Facebook
Sign up using Email and Password
Post as a guest
Required, but never shown
Sign up or log in
StackExchange.ready(function ()
StackExchange.helpers.onClickDraftSave('#login-link');
);
Sign up using Google
Sign up using Facebook
Sign up using Email and Password
Sign up using Google
Sign up using Facebook
Sign up using Email and Password
Post as a guest
Required, but never shown
Required, but never shown
Required, but never shown
Required, but never shown
Required, but never shown
Required, but never shown
Required, but never shown
Required, but never shown
Required, but never shown
Vxp,17KHmYYa,jCn0,JLN5h7 HbUj bkXJD0FLd,Uw